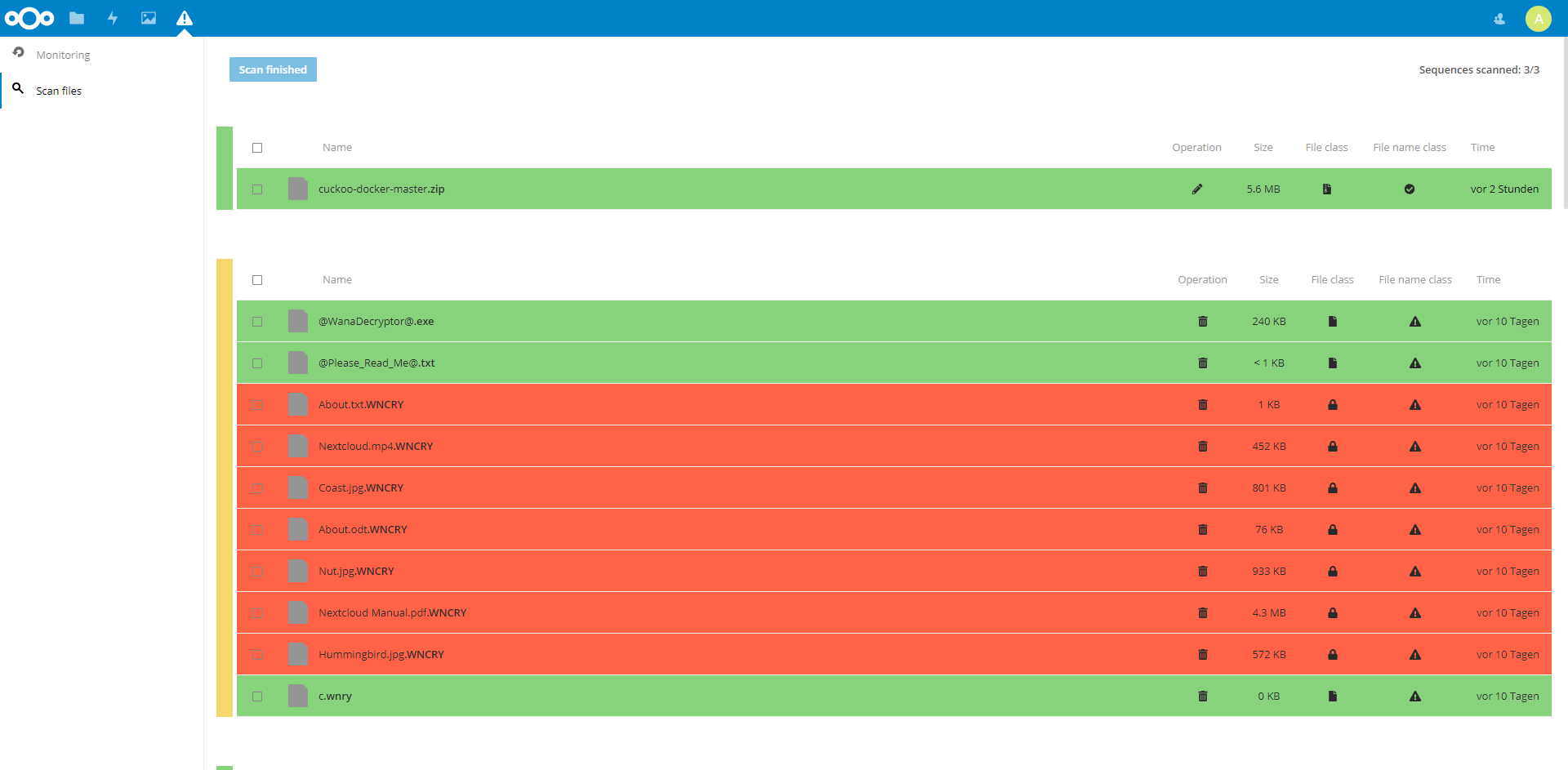
[Master] Ransomware Detection
Ransomware attacks – in contrast to other cyber attacks – must not be detected and especially not blocked or recovered on first sight. This relaxation is supported by the rareness of ransomware attacks. Certainly, the uprising of ransomware families, which are able to circumvent the detection mechanism, integrated into the local machine, prevents the approaches from taking advantage of the relaxation. Justified by the attack isolation, the move to the personal cloud storage is the reasonable way to gain as much as possible of the relaxation, the perfect protection of false positives and the simplified recovery. In this paper we investigate different ransomware families – with the help of real-world malware samples – to formulate a taxonomy of requirements for operation classes and indicator categories. Additionally, we propose an approach which moves the ransomware detection from the local system to the personal cloud storage. Utilizing the file versioning of the cloud storage, we can delay the recovery and reliably perform our content-based, metadata-based and behaviour-based analysis in combination with the `guilt by association' assumption to improve the false positive rate. The new burden for the end-user is the responsibility of supervising the recovery; but the responsibilities are supported by the simplified recovery – offering the classification information – allowing the user to make a qualified decision and release the user from fighting with false classified operations. In summary, this leads to an improvement in the aspects of detection quality, usability and reliable recovery.
You find more information in Detecting Ransomware and the related application.